How Click Fraud Detection Works
Abisola Tanzako | Oct 04, 2024
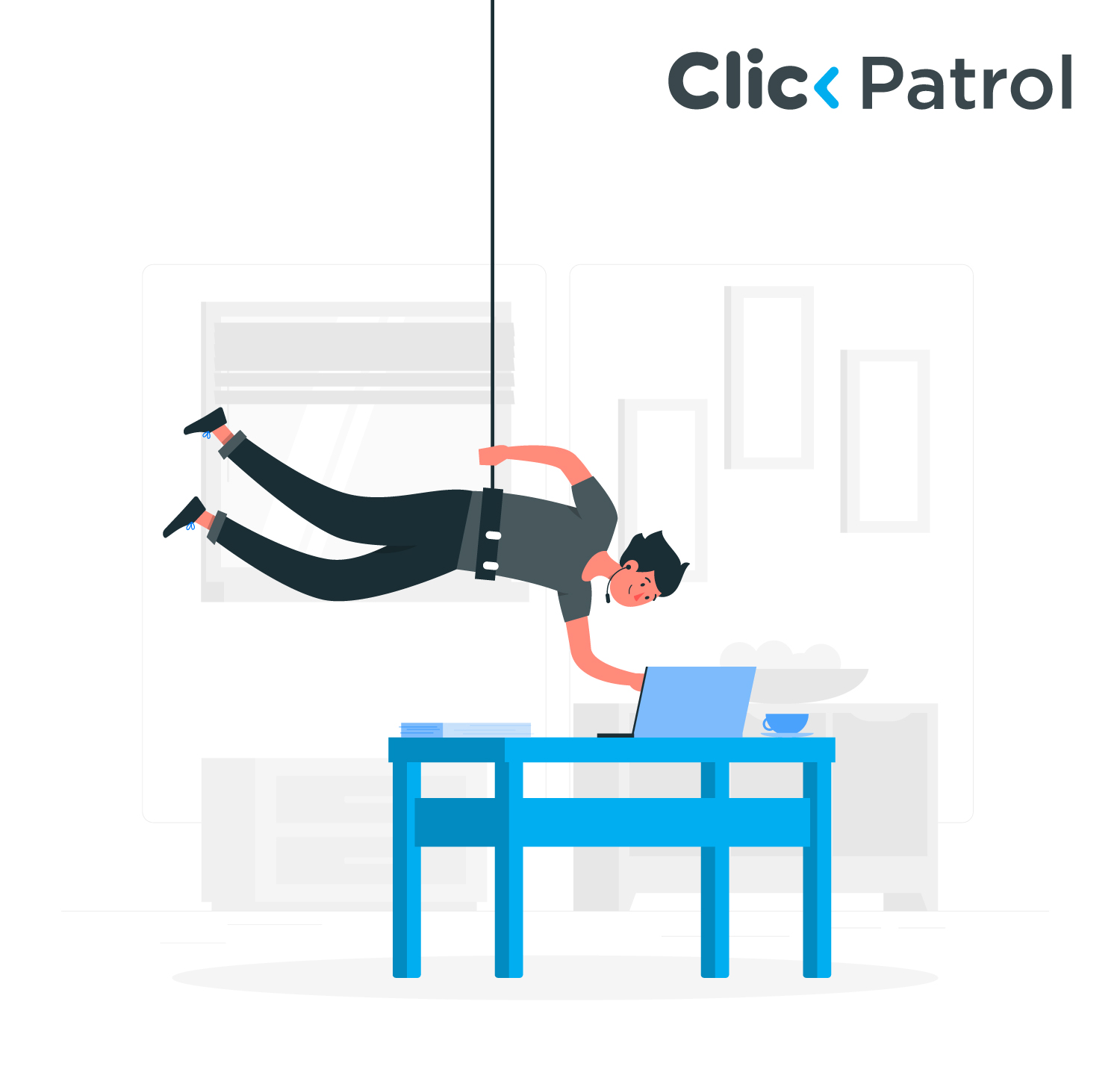
Learning about click fraud detection is essential to keeping your campaigns safe.
Click fraud/fake clicks has now emerged as one of the growing perils of the digital advertising ecosystem. Due to the growing number of businesses taking their advertisements online, fake click activities have also increased. Click fraud entails faking clicks on digital ads by people, automated bots, or competitors without intending to engage with the business. These can cause wasted ad spend, distorted metrics, and lower ROI.
Due to this challenge, companies and advertisers resort to means that would allow them to detect click fraud. To prevent click fraud, businesses employ tools, technologies, and algorithms. This article aims to analyze how click fraud can be detected and measures by which businesses can safeguard their ad against fraudulent clicks.
Understanding click fraud
Fake clicks can be divided into many forms; some of these divisions are:
- Competitor fraud occurs when a competitor clicks on an ad to exhaust the ad budget or reduce the quality of the ad.
- Publisher fraud involves fraud in which the publisher, usually a website owner, merely clicks through ads within their website to increase revenues via pay-per-click systems.
- Bot fraud involves programming automated bots to click on ads repeatedly to simulate the equivalent of human clicks, thereby distorting performance metrics.
These usually do not convert into meaningful engagement and become wasted advertising efforts.
How click fraud detection works
Fake click detection systems have been versatile in combining advanced technologies, machine learning, and human oversight. Some of the approaches to fake click detection are as follows:
1. Data collection and monitoring
Fake click detection starts with gathering data comprehensively; every time a click happens, the system captures quite a few bits of data. These include IP address, device details, geolocation, click pattern, referral URL, and ad display metrics. Such datasets are relevant because they give the fingerprint of users’ behavior, which could further be analyzed to find anomalies or suspicious behaviors.
2. Behavioral analysis
Behavioral analysis is at the forefront of most click fraud detection systems. These systems analyze users’ behavior against expected norms. For example, users usually show natural patterns of scrolling, clicking through to other pages, or at least spending some decent amount of time interacting with the ad content. In contrast, fraudsters or bots, on the other hand, have rapid, repeated clicks and short sessions with no engagement.
This is done by analyzing the generated behavioral data to determine deviation from standard patterns using advanced machine learning algorithms. In other words, the more these systems run, the more effective they become in identifying fraudulent behaviors based on continuous learning, provided the data sets are large.
3. Machine learning algorithms
Machine learning can be considered the backbone of any click fraud detection; this aspect helps in processing bulk data with great speed and recognizes patterns that no human could trace by himself. In machine learning, models can be trained from historical data to understand the difference between fraudulent and legitimate clicks. These models can be categorized into;
Supervised learning would involve training algorithms on labeled data sets comprising legitimate and fraudulent clicks. Thus, the system learns some characteristics from each data set for subsequent usage in new data sets.
Unsupervised learning: Unsupervised models do not need labeled data. They are designed to find an intrinsic structure within the data and try to group clicks into clusters. Anything that looks like an outlier or anomaly is flagged for further investigation.
4. IP filtering and blacklisting
This is one of the most direct methods of detecting and preventing click fraud. This is done by identifying and filtering IP addresses associated with fraudulent activities. This is done through these processes.
- IP monitoring: With every click, an IP address of where it originates from is logged along with it. An abnormal number of clicks emanating from an IP, particularly if made quickly, will be considered fraudulent.
- Blacklisting: This process operates by adding an IP to the list once it has been confirmed to be used or part of fraudulent activity such as a botnet. Any click coming from the blacklisted IP is usually ignored or scrutinized.
IP filtering has become one of the fastest and most efficient ways of blocking sources known to be fraudulent. However, this method is by no means tight, as sophisticated fraudsters may use VPNs, proxy servers, or constantly rotating IP addresses to defeat this form of fraud detection.
5. Device fingerprinting
Device fingerprinting is another key technology in click fraud detection. Unlike IP filtering, which can easily be overcome with proxies or VPNs, device fingerprinting identifies unique attributes connected with the user’s device. These include the following: Browser configurations, operating system versions, installed plugins, screen resolution, language settings, etc.
Since this fingerprint is almost impossible to change, it is one of the most reliable methods of tracking suspicious activity. Even if the fraudster changes his device’s IP address, his fingerprint remains considerably similar. Device fingerprinting significantly raises fraud detection accuracy if applied in addition to other detection methods.
6. Honeypots and traps
A honeypot is a technique for luring and trapping bots or fraudulent click generators. In the context of fake click detection, honeypots are invisible or irrelevant links or ads placed on a website. Legitimate users cannot see or click on these elements because they are hidden or irrelevant. However, automated bots that scan the page will still attempt to click on them, inadvertently outing themselves as fraudsters.
If a click on a honeypot is detected, the system may flag the source as a suspected fraudster. Honeypots are one of the most efficient ways to catch bot traffic; they usually remain undetected. They are also quite useful since they create little to no false positives because legitimate users will not interact with these hidden elements.
7. Analyzing statistics and finding anomalies
Statistical analysis and anomaly detection mechanisms can be used for click fraud detection. Techniques are being applied that may analyze data from clicks to find spikes or trends that are unusual and indicative of fraud. E.g.,
- Click-through rate: An unusual increase in clicks from one IP address, location, or referring site may be fraudulent.
- Conversion discrepancy: Too many clicks and a handful, or none, of conversions can raise a red flag. According to historical data, real users convert at predictable rates.
- Spikes in CTRs: A sudden and unexpected spike in the click-through rate of an ad or campaign signals click fraud, especially if it is not matched or surpassed by a corresponding rise in conversions.
Challenges of click fraud detection
Despite the sophistication of fake click detection systems, challenges are bound to exist. Some of these challenges are;
- Evolving tactics: As digital technology evolves, so do click fraud tactics. Fraudsters make This possible by using advanced tactics like advanced bots, IP rotation, and human-like click behavior to bypass detection systems. Fraudsters continually modify and refine their methods to utilize sophisticated bots, IP rotation, and simulated human-like click behavior that evades detection systems.
- False positives: One of the major challenges of fake click detection is false positives. The system may flag valid clicks as fake, reducing opportunities and ad reach.
- Multi-device usage: Most of the time, users switch to another device in the middle of an internet activity; this can pose a challenge as it makes it difficult to track genuine users.
Insights on click fraud detection
Fake click detection has become a vital part of digital advertising. It allows the advertiser to protect its ad budget, optimize the performance of the campaigns, and ensure that data reporting becomes more accurate. Businesses can detect and prevent click fraud through data collection, machine learning, behavioral analysis, and fraud prevention techniques.
This would allow continuous development of fake click detection systems and make the ad ecosystem much safer and more effective. Click fraud is ever-evolving; however, with the right detection techniques and prevention strategies, businesses can reduce the menace of click fraud to the barest minimum.
FAQs
Q. 1 How does click fraud detection identify fraudulent clicks?
Fake click detection entails tracking IP addresses, click behaviors and metrics, and individual user behavior indicators such as session length and bounce rates. Better tools employ machine learning and AI to analyze traffic to detect peculiarities, identify bots, and differentiate between actual users and scams.
Q. 2 What role does machine learning play in click fraud detection?
Machine learning is beneficial in modern click fraud detection because of the enhanced capability of recognizing patterns in big data and the ability to learn from newer data. These can also detect exceptions, see through new methods of fraud, and predict other fraudulent actions, making them tremendously preferable for actual detection and prevention.
Q. 3 Can click fraud detection tools block fraudulent clicks in real-time?
Yes, many advanced fake click detection tools are developed in real-time to detect and block fake clicks. These tools detect fraudulent activity and are based on data like IP address, geolocation, and click behavior; they help block a fraudulent source before it exhausts the ad budget or influences campaign performance in any way.